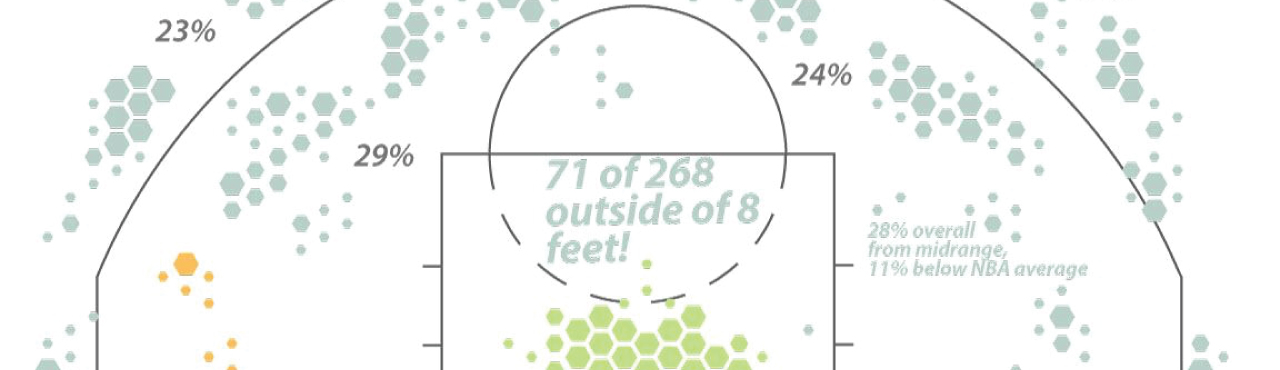
Program
Location: Key 3+4+6
Session 1
8:30-10:10, Session Chair: Marc StreitOpening Address
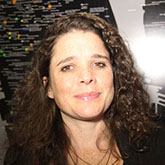
Keynote: Big Data For A Public Good
Sarah Williams, Massachusetts Institute of Technology
Abstract: Big data will not change the world unless it is collected any synthesized into tools that have a public benefit. In this talk Sarah Williams will illustrate projects from her research lab, the Civic Data Design Lab @ MIT, that have transformed data into visualizations that have had an effect on policy reform. From her work on the Digital Matatus project in Nairobi Kenya, where she created the first map of the informal transit system to her work on in on measuring Air Quality in Beijing, to more recent work investigating the Ghost Cities in China. Williams will show how collecting data, visualizing it, and opening up to anyone to use can leverage the power of data to create real policy change.
Bio: Sarah Williams is currently an Assistant Professor of Urban Planning and the Director of the Civic Data Design Lab at Massachusetts Institute of Technology’s (MIT) School of Architecture and Planning School. The Civic Data Design Lab works with data, maps, and mobile technologies to develop interactive design and communication strategies that bring urban policy issues to broader audiences. Trained as a Geographer (Clark University), Landscape Architect (University of Pennsylvania), and Urban Planner (MIT), her work combines geographic analysis and design. Her design work has been widely exhibited including work in the Guggenheim and the Museum of Modern Art (MoMA) in New York City. Before coming to MIT, Williams was Co-Director of the Spatial Information Design Lab at Columbia University’s Graduate School of Architecture Planning and Preservation (GSAPP). Williams has won numerous awards including being named top 25 planners in the technology and 2012 Game Changer by Metropolis Magazine. Her work is currently on view in the Museum of Modern Art (MoMA), New York.
9:20-09:45
What Shakespeare Taught Us About (Visual) Data Science
Michael Gleicher
Abstract: The Visualizing English Print (VEP) has sought to enable literature scholars to exploit large document collections. We have both provided computational tools and thinking to scholars, but have also learned about their approaches, concerns, and ways of thinking. These lessons from literary scholarship can inform our thinking about data science in general, and specifically the roles for visual tools.
Speaker Bio: Michael Gleicher is a Professor in the Department of Computer Sciences at the University of Wisconsin, Madison. Prof. Gleicher is founder of the Department's Visual Computing Group. His research interests span the range of visual computing, including data visualization, image and video processing tools, virtual reality, and character animation techniques for films, games and robotics. Prior to joining the university, Prof. Gleicher was a researcher at The Autodesk Vision Technology Center and in Apple Computer's Advanced Technology Group. He earned his Ph. D. in Computer Science from Carnegie Mellon University, and holds a B.S.E. in Electrical Engineering from Duke University. Prof. Gleicher is an ACM Distinguished Scientist.
9:45-10:10
Explanatory Visual Analytics for Enhancing Human Interpretability of Machine Learning Models
Josua Krause, Aritra Dasgupta, Enrico Bertini
Abstract: The recent successes of machine learning (ML) have exposed the need for making models more interpretable and accessible to different stakeholders in the data science ecosystem, like domain experts, data analysts, and even the general public. The assumption here is that higher interpretability will lead to more confident human decision-making based on model outcomes. In this talk, we report on two main contributions. First, we describe the role of model explanations by providing examples of well-known ML models. Second, we discuss how explanatory visual analytics systems can be instantiated for enhancing model interpretability by referring to our past and current research.
Speaker Bio: Josua Krause is a PhD Candidate at NYU Tandon School of Engineering. His research focus is on the intersection of machine learning and visualization. Recently, he has been working on interpretability and explanation of black-box machine learning models with the help of visual analytics and has been actively publishing in this area. He received his Master degree from the University of Konstanz in 2014.
Session 2
10:30-12:10, Session Chair: Daniel Keim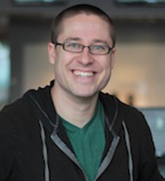
Keynote: Teaching Data Visualization to 4 Million Data Scientists - Lessons from Evidence Based Data Analysis
Jeff Leek, Johns Hopkins University
Abstract: Statistics is at least as much an art as it is a science. Nowhere is this blend more clear than in the area of data and information visualization. One of the key open questions is how datavisualization fits in to the data analytic process and how decisions made by analysts during exploratory data analysis impact downstream statistical models. In this talk I will discuss evidence based data analysis, how we can use large scale teaching platforms to experiment with visualizations, and how we can use this to move the art of data analysis toward a science.
Bio: Jeff Leek is an Associate Professor of Biostatistics and Oncology at the Johns Hopkins Bloomberg School of Public Health. His research focuses on the intersection of high dimensional data analysis, genomics, and public health. He is the co-editor of the Simply Statistics Blog and co-director of the Johns Hopkins Specialization in Data Science.
11:10-11:40
The Role of Visualization in Prediction
Adam Perer
Abstract: There are a growing number of data scientists that wish to go beyond exploring data: they do wish to interpret their data but they also want to know what might happen next. Predictive modeling is becoming a common practice among data scientists to fill this gap, but these techniques are often black boxes that may limit domain experts's contributions to the models. My talk will investigate the role of visualization in prediction. I will discuss systems that we've built to highlight how informative visualizations can assist in all stages of predictive modeling, from cohort selection to feature selection to probing the model to understand why predictions were made. Our research suggests this interpretation can result in more accurate and comprehensible predictions.
Speaker Bio: Adam Perer is a Research Scientist at IBM's T.J. Watson Research Center, where he is a member of the Healthcare Analytics Research Group. His research in visualization and human-computer interaction focuses on the design of novel visual analytics systems. He received his Ph.D. in Computer Science from the Human-Computer Interaction Lab at the University of Maryland. His work has been published at premier venues in visualization, human-computer interaction, and medical informatics (IEEE InfoVis, IEEE VAST, ACM CHI, ACM CSCW, ACM IUI, AMIA). More information about Adam's research is available at http://perer.org
11:40-12:10
Visual Analysis of Hidden State Dynamics in Recurrent Neural Networks
Hendrik Strobelt, Sebastian Gehrmann, Bernd Huber, Hanspeter Pfister, Alexander M. Rush
Abstract: Recurrent neural networks, and in particular long short-term memory networks (LSTMs), are a remarkably effective tool for sequence modeling that learn a dense black-box hidden representation of sequential input. Researchers interested in better understanding these models have studied the changes in hidden state representations over time and noticed some interpretable patterns but also significant noise. We present LSTMVis a visual analysis tool for recurrent neural networks with a focus on understanding these hidden state dynamics. The tool allows a user to select a hypothesis input range to focus on local state changes, to match these states changes to similar patterns in a large data set, and to align these results with domain specific structural annotations. We show several use cases of the tool for analyzing hidden state properties on datasets containing nesting and phrase structure. We demonstrate how the tool can be used to isolate patterns for further statistical analysis.
Speaker Bio: Hendrik Strobelt is a postdoctoral researcher in the Visual Computing Group at Harvard SEAS. He received his PhD in Computer Science from University of Konstanz and his MSc (Diplom) from TU Dresden.
Session 3
2:00-3:40, Session Chair: Hanspeter PfisterPANEL: Teaching Data Science and Visualization: What works, what doesn't?
Jeff Leek, Patrick Lucey, Klaus Mueller, Sarah Williams
Moderator: Hanspeter Pfister
3:00-3:25
Advancing Additive Manufacturing Through Visual Data Science
Chad Steed, Ryan Dehoff, William Halsey, Sean Yoder, Vincent Paquit, Sarah Powers
Abstract: As advances in large-scale 3D printing spark revolutionary ideas in additive manufacturing, visual data science is adding fuel to the fire. Technological leaps in this field have given designers an unprecedented degree of geometrical freedom for building complex objects with minimal waste material. The key to realizing the full potential of large-scale 3D printers lies in acquiring a deep understanding of the printing process using a visual data science approach. To this end, additive manufacturing researchers at the Oak Ridge National Laboratory Manufacturing Demonstration Facility have teamed with visual analytics researchers to develop a new visual analytics system, called Falcon, that allows interactive, multi-scale hypothesis formulation and confirmation for 3D printer log files. In this talk, we will present the interactive data visualization techniques in the Falcon system with an emphasis on examples involving real data analysis scenarios with additive manufacturing experts.
Speaker Bio: Dr. Chad A. Steed is a Senior Researcher in the Computational Data Analytics Group at the Oak Ridge National Laboratory (ORNL) and he holds a Joint Faculty Appointment with the University of Tennessee's Electrical Engineering and Computer Science Department. He received the Ph.D. degree in Computer Science from Mississippi State University, where he studied visualization and computer graphics. His research spans the life cycle of data science including interactive data visualization, data mining, human-computer interaction, visual perception, databases, and graphical design. Dr. Steed's work is currently focused on designing systems that combine automated analytics with interactive data visualizations to enhance human exploration and comprehension of complex data. He is the recipient of the 2014 UT-Battelle Early Career Researcher Award, a 2014 ORNL Technology Commercialization Award, a 2013 R&D 100 Award, and a 2013 ORNL Technology Commercialization Award.
3:25-3:3:40
Clusterix: a visual analytics approach to clustering
Eamonn Maguire, Ilias Koutsakis, Gilles Louppe
Abstract: Data clustering is a common task in many data science workflows where we wish to gain insight into the sub groups that exist within a data set. However, there are numerous clustering algorithms, and parameters. Coupled with the variability in the input data, the interdependencies between features, and the task at hand, it is a rare occurrence to find meaningful results in your data immediately. Data scientists will frequently tune parameters, drop or add features (and derive new ones) to find meaningful clusters in their data. Clusterix is a visual analytics tool created to support users in such tasks. Clusterix was primarily developed to support the task of text clustering, in particular the clustering of institution strings from publications for the INSPIREHEP repository based at CERN. However, it has been generalized to support all types of data.
Speaker Bio: Eamonn completed his DPhil (PhD) at the University of Oxford in computer science, focused on data visualization, in particular the systematisation of glyph design. He joined CERN as a Marie Curie COFUND Fellow in 2015 where he leads development of the new hepdata.net platform and contributes to numerous other visualization and data projects at CERN. Previously, he was the lead software engineer at the Oxford University e-Research Centre, where he led development of bioinformatics tools and a visual analytics platform for corporate insider threat detection. His research interests are in the merging of machine learning and visual analytics.
Session 4
4:15-5:55, Session Chair: Alexander Lex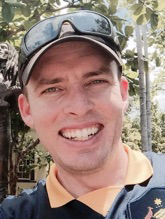
Keynote: Interactive Sports Analytics: Going Beyond Spreadsheets
Patrick Lucey, STATS
Abstract: Imagine watching a sports game live and having the ability to find all plays which are similar to what just happened immediately. Better still, imagine having the ability to draw a play with the x’s and o’s on an interface, like a coach draws up on a chalkboard and finding all the plays like that instantaneously and conduct analytics on those plays (i.e., when those plays occur, how many points a team expects from that play). Additionally, imagine having the ability to evaluate the performance of a player in a given situation and compare it against another player in exactly the same position. We call this approach “Interactive Sports Analytics” and in this talk, I will describe methods to find play similarity using multi-agent trajectory data, as well as predicting fine-grain plays. I will show examples using STATS SportVU data in basketball, Prozone data in soccer and Hawk-Eye in tennis.
Bio: I have recently accepted the position of Director of Data Science at STATS. Previously, I was an Associate Research Scientist at Disney Research Pittsburgh, where I conducted research into Group Behavior and Sport Analytics. My research centers on representing, learning and predicting both cooperative and adversarial groups using spatiotemporal data - with application to continuous sports and audience domains. Before that, I was at the Robotics Institute at CMU as well as at the Faculty of Psychology at the University of Pittsburgh where I conducted my research on using facial expressions to aid in the diagnosis of medical conditions (such as Pain, Depression and Facial Paralysis) with Prof. Jeff Cohn.
4:55-5:10
Coupled Interactive Visualization and Machine Learning for Accelerated Model Development: Applications to Electronic Healthcare Record Data
Charles Fisher, Randall Frank
Abstract: Modern machine learning frameworks provide unprecedented methodologies capable of modeling hundreds of latent factors buried in complex datasets. One goal of the application of these methods to EHR data is to synthesize predictive models that can be effectively applied to simple subsets of input data. Unconstrained, automated methods for developing these models often provide results, which are self-fulfilling or physiologically nonsensical. Here we present a collection of visual tools designed to support semi-automated methods for EHR model generation that facilitate the integration of domain expert knowledge and decision support. The talk will focus on pitfalls encountered in the development of specific models and key insights the tools provided during their application to a historical cohort of 1000 burn patients at the San Antonio Military Medical Center (SAMMC).
Speaker Bio: Charles Fisher is a scientist at Applied Research Associates, Inc., where he researches and models weapons effects for the Integrated Munitions Effects Assessment (IMEA) software. Mr. Fisher also works in ARA's decision support team developing statistical based ML prediction tools for the Army Institute of Surgical Research. Further, he is currently leading a project developing dynamic models of cerebral blood flow following a traumatic brain injury event. Mr. Fisher recently collaborated on conference manuscripts on urban airblast effects for the 16th International Symposium for the Interaction of the Effects of Munitions with Structures and on ML health care prediction tools for the 15th International Conference on Complex Acute Illness. In 2009 Mr. Fisher began his engineer career with live testing and modeling of IED blast events for personnel mitigation purposes. He received his M.S. in Applied Mathematics from Worcester Polytechnic Institute in 2013. He can be contacted at cfisher@ara.com.
5:10-5:25
Data Shading: Building Data Models from Visualizations
Joseph Cottam, Andrew Lumsdaine
Abstract: Visualizations are data models. They capture some elements of the underlying data, while obscuring others. Unlike most data models, visualizations are typically consumed directly by a person using visual perception. In contrast, most data models are used as inputs into another analysis step. With a small change to how a visualization is produced, applying a process we call ``data shading'', a visualization can also be used as data model and fed into further analysis steps. This abstract gives a brief summary of data shading and illustrates one of its applications. Using a visualization as a data model directly enriches the analytical process by providing a unique pairing of algorithmic and human perceptual analysis.
Speaker Bio: Dr. Joseph Cottam works on visualization frameworks for out-of-core and streaming data scenarios. He is working on improving the interplay between data analysis and visualization. His interested include visualization, data analysis and programming languages. His work has touched on streaming data, high performance computing and out-of-core methods. He is one of the architects and developers for Bokeh (a python visualization tool). He is currently a research associate at Indiana University.
5:25-5:40
Causal Inference in Time Series Data Using Autoencoder
Kozen Umezawa, Yosuke Onoue, Hiroaki Natsukawa, Koji Koyamada
Abstract: Visualizing causal relations from time series data is necessary to understand and analyze phenomena. We present a novel causality visualization method to support making a new discovery from large-scale data. In addition, we propose a new idea of causal inference using an autoencoder. We demonstrate that a unit which reacts to causality can be extracted from the autoencoder network. By using other causal inference methods, we show that the output of this unit is proportional to the magnitude of causal relations between two variables. Our causality visualization uses colors to represent the value of the output. To demonstrate the effectiveness of our visualization, we test an ocean dataset. Causal relationships among salinity, temperature and flow velocity are tested. Our results show that causal inference using the autoencoder is an effective approach for spatio-temporal data.
Speaker Bio: Kozen Umezawa is a master's student in the Visualization Lab at Kyoto University in Kyoto, Japan. His research interests include visualization causality, machine learning.
Closing